Written by Helen Barlow, Data Scientist at Evergen
What is AI?
Artificial Intelligence (AI) is everywhere – we use it every day. When you search on Google, browse Netflix for something to watch, or check your weather app to decide if you need to take an umbrella, AI is working in the background to predict, recommend and optimise. While recently Large Language Models (LLMs) such as ChatGPT have been in the spotlight, it is specialised AI developed to focus on single, specific tasks that is the real powerhouse behind the applications we use every day.
AI can be as simple as a ‘rules engine’ that follows a series of logical yes/no questions, or more complex computational models and algorithms used in applications for natural language processing and computer vision, which have been around for decades. These approaches are limited as they can only follow their programming and can become inefficient very quickly. Alternatively, Machine Learning (ML) models can derive information from data allowing them to identify patterns and make predictions or decisions without explicit programming. Machine Learning models are dynamic, with additional high-quality ML models that can learn and evolve for more flexible and effective problem-solving.
How is AI used in the energy sector?
Energy is an essential service with a huge number of parts that need to be well orchestrated for the system to operate reliably. With progress towards Distributed Energy Resources (DERs), AI and Machine Learning have emerged as a transformative force, revolutionising traditional practices and contributing to the ongoing shift towards sustainable and efficient energy solutions.
Some of the uses of AI, both traditional and emerging, are outlined below:
Energy Forecasting
Electricity grids require supply and demand to match closely to keep network frequency within a certain range. Governing bodies run AI models to predict energy demand using historical data, weather patterns, and other relevant factors. These predictions are made available to generators who bid to supply energy to the market.
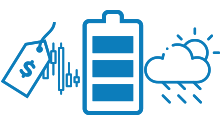
Energy Trading
Algorithms analyse market trends, news, and other relevant data to make informed trading decisions. Price predictions are made by market operators, generators, and retailers at regular intervals throughout the trading period. This trading activity ultimately determines the price of electricity for end consumers.
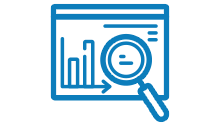
Predictive Maintenance
AI is used for predictive maintenance of energy infrastructure, such as power plants and wind turbines. AI systems can predict when equipment is likely to fail, allowing for proactive maintenance and minimising downtime. Solar farms suffer from less output as their PV panels get dirty and less efficient over time. They can increase their profits by considering maximum generation volumes, actual volumes and costs involved with cleaning to build models that create an optimised cleaning schedule.
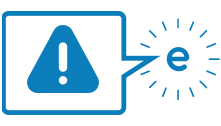
Energy Storage Management (EMS)
AI helps in optimising the operation of energy storage systems, such as batteries, and other controllable loads such as EVs and heat pumps. It can predict energy demand, solar generation and price, and dynamically control the charging and discharging of batteries to minimise costs to the asset owner.
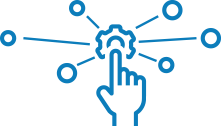
Smart Grids
AI is employed in smart grids to enhance the efficiency and reliability of electricity distribution. It helps in predicting demand, optimising energy distribution, and identifying and managing faults or outages.
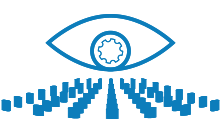
What are the problems our data science team are solving
We work with many different types of clients, from individual sites to VPPs, including commercial operations with a variety of configurations.
In the residential space we have been using AI to optimise sites with batteries and solar generation for many years. Our optimisation technology works by predicting load and solar generation for a site using weather forecasts and historical data which is then optimised against the customer’s electricity tariffs to develop a plan to charge and discharge the battery that will minimise the cost of electricity.
We are developing technology to concurrently optimise hot water heating, EV charging, pool pumps and other controllable devices behind-the-meter. This multi-asset approach is beneficial not just to the customer but also allows greater flexibility for a site to participate in services that stabilise the grid, leading to a more robust and reliable network.
In addition to this, we are working on microgrids – small groups of co-optimised houses. Some of these networks have sites with solar and batteries providing energy at a reduced cost to others in the network, while some have many sites that all have access to one large battery that supports the whole community.
Our technology supports larger commercial sites that have different requirements and control options. With better management over demand, ramp rates, throughput and various configurations of battery and solar available, these sites use custom solutions with site-specific algorithms developed from the building blocks of our optimisation technology. Our modular approach makes it easy to design a solution tailored to each combination of systems quickly and with confidence it will produce the best outcome possible for the site.
What is the future of AI in the energy sector?
As the electricity network moves to a renewables-powered, decentralised grid, AI will become even more important. Grid stability will no longer be about generators increasing supply or switching off to maintain the balance of supply and demand, but rather batteries, EVs, and other devices working in sync to ensure energy gets where it needs to be, when it needs to be there.
From predictive analytics improving energy demand forecasting to smart grid technologies optimising distribution networks, AI plays a pivotal role in enhancing decision-making processes and overall system efficiency. As the energy sector continues to evolve, the strategic implementation of AI-driven solutions becomes increasingly indispensable for achieving a resilient and sustainable energy future.